4 BIG Challenges Prevalent in Speech Recognition Today
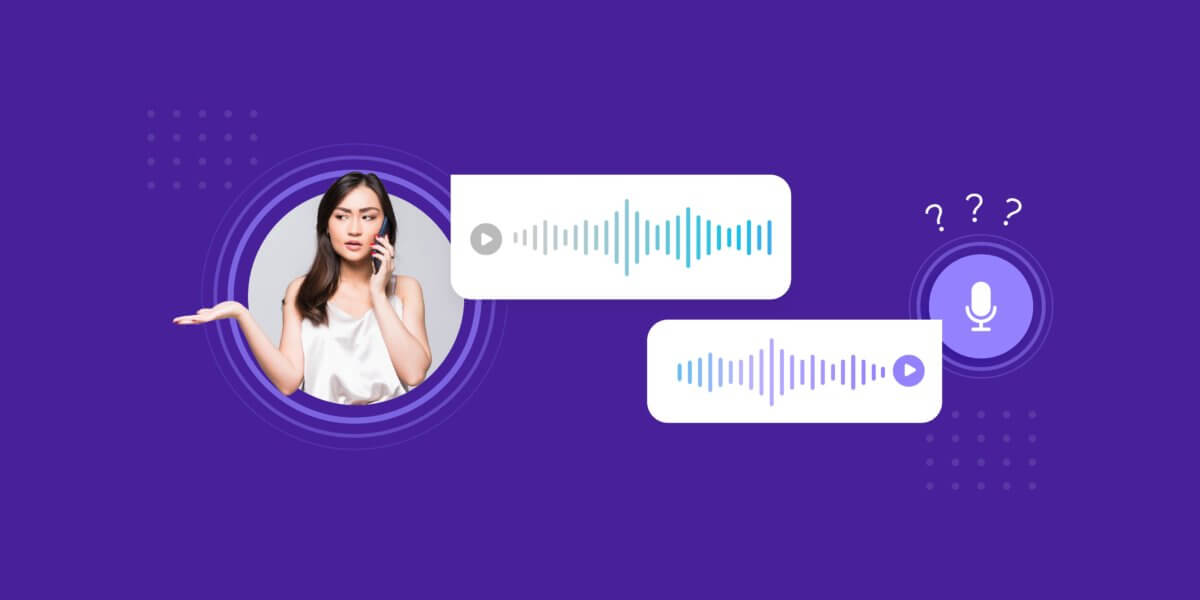
4 BIG Challenges Prevalent in Speech Recognition Today
Speech recognition is difficult to implement in real-time environments. We list the problems that have to be tackled in speech-to-text conversion and solutions for them.
I’m sorry, can you repeat that?
Could you repeat what you just said to me, I didn’t understand.
Sorry, I didn’t quite catch what you said. Can you say that again?
You must have heard your voice assistant ask you that, or a variation of it, on multiple occasions. Or worse, they just go quiet on you.
Voice recognition technology has come a long way since the concept came to light in the 1950s. Over time, users have one persisting problem with voice recognition, and that is accuracy.
No wonder 73% of businesses believe lack of accuracy is the key reason why they don’t use voice technology. This is why building AI algorithms that accurately process voice input has been consistently focal in the R&D of speech recognition.
And why wouldn’t that be? Even with barriers to adoption, voice AI is highly popular among digital consumers today. As many as 65% of users between the age of 25-49 speak to their voice-enabled devices every day.
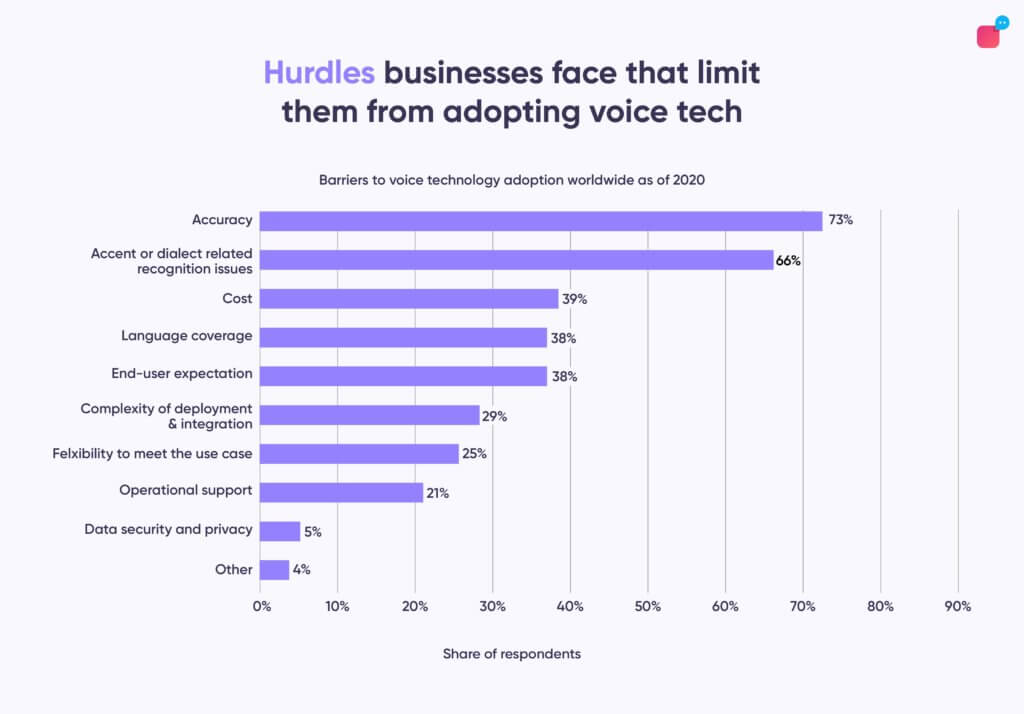
Look at speech as the fastest way to jump over the clutter to arrive at the right spot in the shortest time possible. This is truly what most users today want and expect – to arrive at the right place at the right time. Indeed, automatic speech recognition (ASR) holds the key to amazing experiences brands may be missing to deliver to the users in the post-pandemic world.
4 challenges you will face building your automatic speech recognition system (ASR)
Though we know the potential ASR holds, building an algorithm that’s highly accurate and intuitive, while no impossible knot, may still be a tough nut to crack. So, what are the top ASR challenges you may face when adopting the tech?
1. Lack of lingual knowledge
What makes speech recognition difficult is the lack of language training.
Companies often seem to overlook the fact that English is not the universal language. So expecting users from different geographies to have the same level of proficiency is unrealistic. In fact, 38% of users are hesitant to adopt voice technology because of AI’s language coverage.
If you are trying to deploy your voice assistants in a location, the ASR will likely tank if not trained on specific language models of the region. And even when it is trained for the language, another challenge for ASR is the ability to differentiate between varying dialects and accents for more accurate interpretation.
For example, a user who needs groceries may say “Buy vege-table” to the voice assistant, pronouncing the word a bit differently than the widely accepted “veg-tible” – also the only one AI is familiar with. A poorly trained bot may mistake this input as “buy a veggie table” assuming the speaker wants to buy a table – Highly inaccurate!
2. Peripheral background sounds
Another top speech recognition problem that needs a solution is – noise. It is everywhere! And so, it becomes the job of the ASR solution to accurately catch the speech input through unwanted sounds. An ASR should be able to pick up the input’s sound waves even from a distance in a room riddled with white noise and cross-talk. Echo, for example, also adds to the imprecision. Reflected sound waves from surfaces in the space distort the receptor’s ability to process the actual input unerringly.
3. Low data reliability of ASR
What are the other challenges of speech recognition? Data privacy. While we are making progress in the field of AI, many users are still hesitant to use ASR bots to handle tasks that involve sensitive data and money. Data privacy is sovereign to users who wish to exercise some level of governance and transparency with their information.
PWC says that one of the three main reasons why users are scared to experiment with voice tech is simply a lack of trust. Where more than half of the users use their voice assistant to buy online, all of these purchases are trivial with low spending. And so, data concerns remain the challenges and issues businesses face in adopting speech recognition technology. Users don’t trust voice assistants as much, so businesses must be prepared to face reluctance in adoption from their market.
Costs and deployment
Other reasons why speech recognition is difficult to implement in real-time environments are because of the capital and infrastructure needed.
Implementing an ASR system needs a far-sighted vision. It’s a long game and not a change that occurs overnight. Bearing this in mind, you need to be prepared to handle the time, resources, and capital involved in building, testing, and deploying the system in the market. For example, the lack of visual elements makes designing interactive voice user interfaces (VUIs) more complex than designing UI for chatbots.
Another disadvantage of speech recognition can be that training language models take considerable time and expertise. Gathering enough language resources or effectively making do with the available ones may not come cheap. All in all, manual development would rain heavily on your pockets.
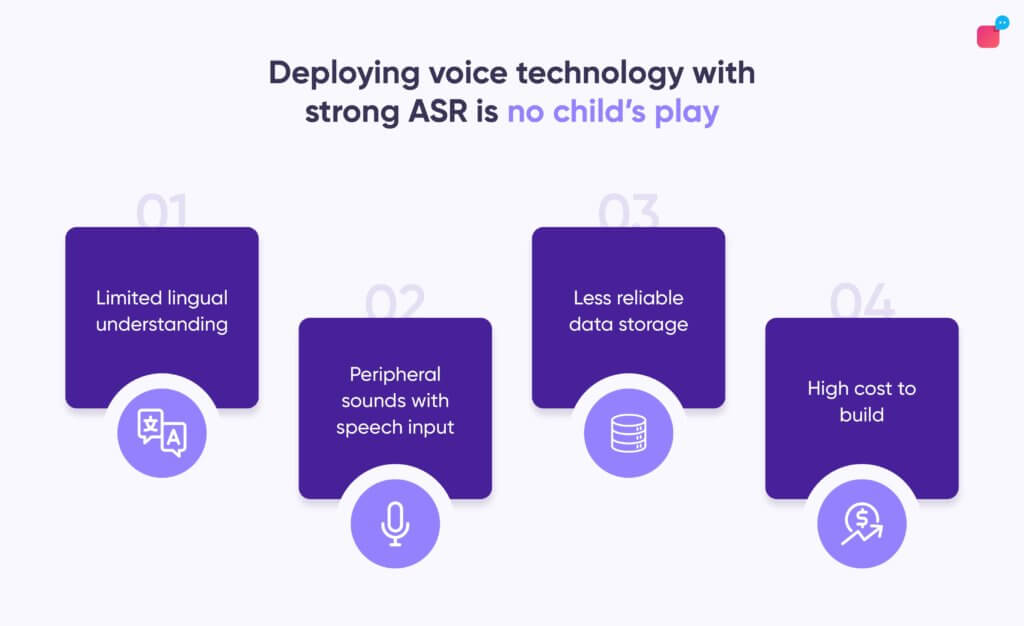
How can you overcome challenges and issues in adopting speech recognition technology?
Implementing an automatic speech recognition system today is a sure-shot way to stand out from the crowd that still uses outdated ways.
With new possibilities, come challenges. Turning the disadvantages of speech recognition into your strengths requires work. For example, one way to train your ASR to perform well is by seeding it through non-ideal training environments and reducing noise from the audio input before speech-to-text (STT) conversion.
Where user intent and localisation come into the picture, businesses need to focus on specific regions. Ideally, an ASR model pre-trained on multiple intents and languages with a focus on dialects/accents is a win-win. The speech recognition system identifies the language regardless of the speaker’s accent or place of origin (dialect differs).
Just like what Voice by Verloop.io does! State-of-the-art ASR by Verloop.io is custom-trained on 1000s of hours of voice data and natively supports 20+ languages (in all shapes, forms, and sizes!) to ensure accuracy at an affordable cost. Despite this, noise suppression and reduction pose a key challenge for voice technology adopters. To ensure you don’t put your user privacy at risk, researching the security protocols ASR providers follow is a great start.
Learn how Verloop.io Improved its ASR accuracy with error correction techniques here.
In conclusion
Speech recognition is an evolving technology, with improvements refining how it functions every day. While these 4 are the key challenges you will come across when building an ASR solution, other factors may also hinder its growth. Lack of utterances, disorganised speech, or simply machine errors can also impede ASR development.
Voice by Verloop.io offers leading speech technology for customer support teams. Fortified with proprietary NLP and spoken language understanding (SLU), our voice technology offers support to people as, when, where, and however they need it.
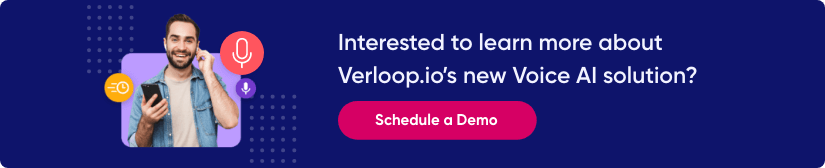