3 Questions to Ask When Someone Says Their Chatbot is “AI-Powered”
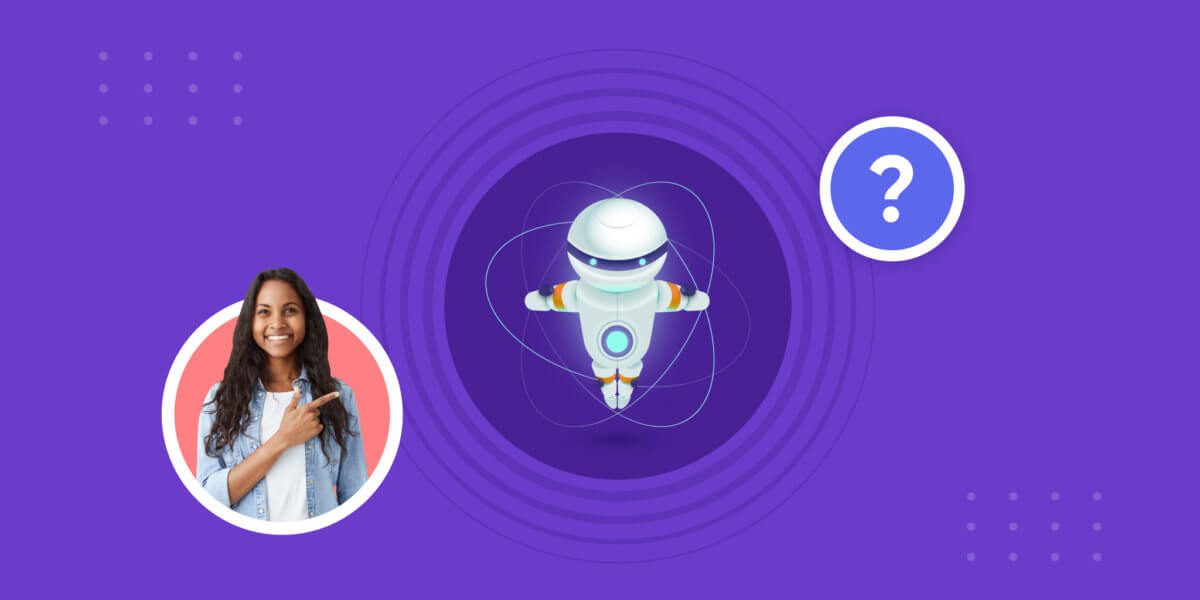
3 Questions to Ask When Someone Says Their Chatbot is “AI-Powered”
AI-powered chatbots are the craze. But is the chatbot powering your customer communication really powered by AI? Here’s how you can find out.
Findings from the 2021 McKinsey survey indicate that AI adoption is continuing its steady rise: 56 per cent of all respondents report AI adoption in at least one function, up from 50 per cent in 2020.
Catchy headlines like these make it easy to wonder if your enterprise firm is doing enough to keep up with the competition. But how do you cut through the noise?
AI is an incredibly broad and evocative term. Its sole purpose in media today is often simply to rally the masses in one direction or the other. So let’s take it from the top. Let’s start off with that report. Where are these enterprise companies applying this AI?
According to the same report, the top three use cases of AI are
- service-operations optimization,
- AI-based enhancement of products, and
- contact-centre automation.
So when a sales rep says their chatbot or any product that they’re trying to pitch to you is an AI-powered chatbot, a few intelligible questions will take you a long way.
By the end of this article, you will know:
- What is an AI-powered chatbot?
- How does an AI-powered chatbot work?
- What 3 questions to ask when someone says their chatbot is ‘AI-powered’?
What is an AI-powered chatbot?
An AI chatbot is one that uses AI technologies such as NLP, ML, and advanced analytics to respond to user queries intelligently and naturally. In simple words, AI-powered chatbots understand human language (text or voice data) and respond just like humans do.
In chatbots, AI is in the form of conversational AI and comprises the following components:
- Machine Learning
- Natural Language Understanding
- Automatic Speech Recognition
- Text-to-Speech and Speech-to-Text
In comparison to other chatbot types (e.g. rule-based and hybrid) AI-powered chatbots understand context and self-learn from new information. With the right integrations, they are able to personalise customer interactions and improve their experience.
How does an AI-powered chatbot work?
AI-powered chatbots use a multi-step process to produce the end result: intelligent and natural conversation. Broadly speaking, the process is divided into four steps:
Step 1: Input Generation
The user inputs their query through a messaging platform such as website chatbots, WhatsApp, Facebook, Viber, etc.)
Step 2: Input Analysis
The AI uses NLU and NLP to break down the text into smaller parts and pull meaning out of the words. Once the machine understands the input, the ML engine analyses the content to understand the intent behind the query.
Step 3: Output Generation
Now that the AI has understood the user’s question, it will match the query with a relevant answer. With the help of natural language generation (NLG), it will respond to the user. Before generating the output, the AI interacts with integrated systems (the businesses’ customer databases) to go through the user’s profile and previous conversations. This helps in narrowing down the answer based on customer data and adds a layer of personalisation to the response.
Step 4: Reinforcement Learning
This is where the self-learning part of a conversational AI chatbot comes into play. Based on how satisfied the user was with the answer, AI is trained to refine its response in the next interaction. With each interaction, businesses get a treasure trove of data full of variations in intent and utterances which are used to train the AI further. Over time, the user gets quicker and more accurate responses, improving the experience while interacting with the machine.
What 3 questions to ask when someone says their chatbot is ‘AI-powered’?
Now that we know how AI works in a chatbot, let’s find out what questions will help you get closer to answering “Is a chatbot AI-powered?”
Suggested Reading: Customer Support Automation and Its Importance
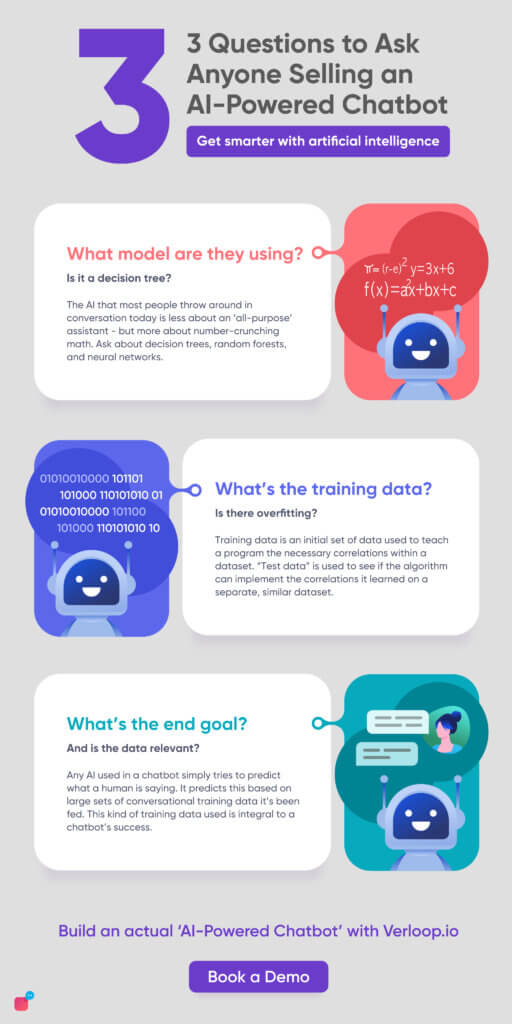
1. Can you explain what AI-powered chatbot model you’re using?
Let’s start off with the big disclaimer: Any AI product is only as powerful as the model that powers it. AI-powered chatbot comes to the next level of automation.
As we mentioned earlier, there are a lot of eye-catching headlines in the world. But in (an admittedly oversimplified) reality, terms like AI, ML, Predictive Analysis and Neural Networks are just variations of the same core ideology that’s existed since the 1950s.
The “AI” that most people throw around in conversation today is less about an all-purpose assistant – but more about number-crunching math.
And that’s where statistical models kick in.
Decision trees are often the most common ones around – and should be your first line of questioning.
IS IT A DECISION TREE?
Decision trees are produced by algorithms that identify various ways of splitting a data set into branch-like segments. These segments form an inverted decision tree that originates with a root node at the top of the tree.
A decision tree is fairly easy to understand and is beloved for that very reason. An empathetic data scientist could easily walk you through the branches of his work.
A ‘random forest’ or ‘neural network’ requires a more scientific background. But asking whether it’s a decision tree model (and how the model works) is often enough to stump most people who are puffy-chested salesmen.
2. Can you talk to me about your training and test data?
Machine Learning is based on two sets of data: Training data and test data.
Understanding what each one is and what they do gives you incredible leverage in conversations with braggadocious salesmen who boast about their AI-powered products that give “100% accuracy and precision”.
Training data
Assume you’ve taken half the population of a small town as a sample set. You’ve collected all necessary demographic data-name, age, gender, income, ethnicity, religion, and family structure.
Your goal is to try to figure out how many of them grow up to like the colour blue.
Since you have all the required details – name, age, etc., you can build a model to identify correlations and trends. You find that a certain demographic group is more likely to like blue when compared to their peers.
This sample set that you’ve used to teach the model is called training data.
“Training data” is an initial set of data used to teach a program the necessary correlations within the dataset.
You now have a model to predict who likes blue. Time to put it to the test.
Test AI-powered chatbot data
You now use the other half of the town’s population. This is the test data.
“Test data” is trying to see if the algorithm can implement the correlations it observed during training on a separate, similar dataset.
If the model can successfully predict who likes blue amongst the second half of the town’s population based on what it learned from the first half during training, the model is doing its job well.
This part of the process is where most marketers and salesmen get their “close to 100% accurate” claim. But there’s a lot that can go wrong with this number. Case in point – overfitting.
Suggested Reading: How to Build a Chatbot?
3. How is the training data relevant to your end goal?
An AI-powered chatbot’s end goal is to simulate human conversation with its customers, clients, and visitors.
Any AI used in a chatbot is simply trying to predict what a human is saying. It predicts this based on large sets of conversational training data it’s been fed.
This makes the kind of training data that is used extremely important to a chatbot’s success.
Taking our analogy into consideration – while gender and age are likely important qualifiers to determine whether someone will go to college, it is unlikely that a variable like hair colour or preference for soft drinks has any standing in higher education.
You want to remove all potential noise from your training data to ensure clean results. Not doing so is called “data dredging”.
It’s also important to note that the predictions that an AI-powered tool presents are correlation – not causation.
AI-powered chatbot: future of business-customer communication
If you’re curious to see how an actual AI-powered chatbot works and want to deploy one for your customer interactions, then you are in the right place. Sign up for a demo and our experts will take you through the product, the AI and different use cases customised for your business. We’ll answer any questions you may have.